Multi-fidelity Fusion and Optimization Theory and Applications
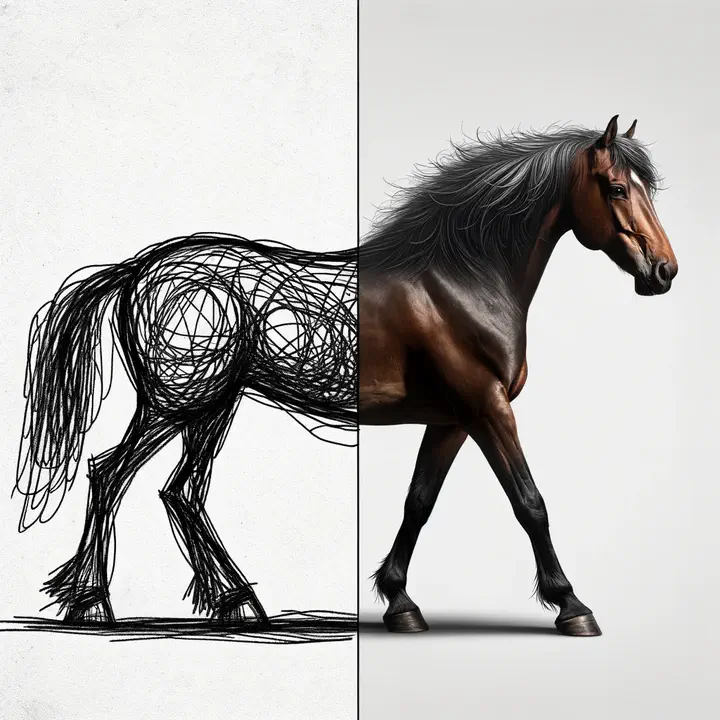
University of Sheffield Collaborating Faculties: Faculty of Science, Faculty of Engineering and AMRC
Overview: Our project aims to investigate novel methods to leverage a special type of multimodal data—multi-fidelity data—to improve AI model accuracy and efficiency, leading to scalable solutions in computationally intensive optimisation problems in various engineering disciplines.
Motivation: Our goal is to create innovative techniques and toolsets capable of assimilating and being able to use multi-fidelity data effectively (i.e., providing the same outputs of high-fidelity datasets using lower-fidelity datasets), such as simulation outcomes from both coarse and dense meshes, and signals from high-cost precision sensors and low-cost basic sensors. We aim to enhance AI model accuracy without relying on highly accurate data obtained from high-cost precision sensors only, whilst improving efficiency metrics such as training time and memory cost. We will implement the proposed method in simulation-based testing for autonomous driving systems (ADS) to demonstrate the capability for the safety and reliability of self-driving cars with low-cost computation.